Selecting National Football League pass catchers without a predetermined strategy, prioritizing specific attributes, or considering team needs creates an unpredictable group. For instance, a computer program could generate a list that includes a veteran star, a promising rookie, and a journeyman practice squad player. This approach emphasizes the vast pool of talent and diverse skill sets within the league’s receiving corps.
Unbiased selection offers valuable insights. Analyzing performance data from such an assortment can reveal hidden trends and challenge conventional wisdom regarding player evaluation. Historically, talent evaluation has relied on scouting reports, combine metrics, and draft position. However, a randomized approach offers a fresh perspective, potentially uncovering undervalued players or revealing statistical anomalies. This method can also be utilized for fantasy football drafting, mock drafts, or simply exploring the breadth of receiving talent across the NFL.
The following sections will delve deeper into specific applications of unbiased player selection, exploring statistical analysis techniques and demonstrating how these methods can be applied to various contexts within professional football. Furthermore, the discussion will expand to consider the implications of these findings for team building, player development, and the overall evolution of the game.
1. Unpredictability
Unpredictability forms the foundation of randomly selected NFL wide receivers. This inherent randomness ensures that pre-existing biases regarding player reputation, team affiliation, or perceived potential do not influence the selection process. Cause and effect are directly linked: the random selection method causes an unpredictable outcome, a diverse group of players. This unpredictability is crucial for objective analysis and allows for the discovery of unexpected insights. For example, a random selection might include a relatively unknown player with exceptionally high yards-after-catch compared to a highly drafted but less effective counterpart. Such a comparison, facilitated by the element of unpredictability, challenges established hierarchies and promotes a more nuanced understanding of receiver performance.
The importance of unpredictability extends beyond individual player comparisons. By analyzing a randomly selected group, one can gain insights into broader league trends. Examining the average yards per reception of a random group versus a group of Pro Bowl receivers can highlight the overall distribution of talent within the league. This approach also minimizes the impact of outlier performances that might skew results in smaller, curated samples. Imagine analyzing the impact of specific offensive schemes; a random sample of receivers provides a more representative cross-section of the league, offering a more accurate reflection of scheme effectiveness.
In conclusion, unpredictability serves as a critical component of random NFL wide receiver selection. It ensures objectivity, enables the discovery of hidden talent, and facilitates more accurate analysis of league-wide trends. While traditional scouting methods often focus on predictable metrics and projected potential, embracing unpredictability allows for a deeper, data-driven understanding of receiver performance and its various contributing factors. This approach presents challenges to conventional player evaluation but offers significant potential for uncovering valuable insights and improving talent identification within the NFL.
2. Statistical Analysis
Statistical analysis provides a powerful framework for interpreting the performance of randomly selected NFL wide receivers. By applying statistical methods to data from a diverse and unpredictable group of players, analysts can uncover hidden trends, challenge conventional wisdom, and gain a deeper understanding of receiver performance beyond traditional scouting reports and subjective assessments.
-
Performance Metrics Distribution
Examining the distribution of key performance metrics, such as receptions, yards, and touchdowns, across a randomly selected group of receivers provides valuable context. This analysis reveals the range of performance levels within the league and identifies outliers. For instance, comparing the average yards after catch of a random group to the league average illuminates the relative effectiveness of different receivers in generating yards after the catch. This approach offers a more nuanced understanding of player performance compared to simply ranking players based on total yardage.
-
Correlation Analysis
Correlation analysis explores relationships between different variables within the randomly selected group. Analyzing the correlation between a receiver’s height and their average contested catch rate can reveal whether taller receivers are indeed more effective in contested catch situations. This data-driven approach provides empirical evidence to support or refute commonly held assumptions about player attributes and their impact on performance. Such insights can inform future draft strategies and player development programs.
-
Regression Modeling
Regression modeling allows analysts to predict a receiver’s performance based on a set of predictor variables. Using a randomly selected group of receivers, one can build a regression model to predict a receiver’s future touchdown production based on factors like past performance, draft position, and combine metrics. The randomness of the sample helps ensure that the model is not biased towards specific player types or team contexts. This approach provides a more objective and robust prediction model compared to relying solely on expert opinion.
-
Hypothesis Testing
Hypothesis testing allows for rigorous examination of specific claims about receiver performance. For example, one might hypothesize that receivers from certain conferences are more likely to succeed in the NFL. By analyzing a randomly selected group of receivers, researchers can test this hypothesis and determine whether there is statistically significant evidence to support it. This approach ensures that conclusions are based on data rather than anecdotal evidence or preconceived notions.
By applying these statistical methods to a randomly selected group of NFL wide receivers, analysts can gain objective and nuanced insights into player performance, league trends, and the effectiveness of different talent evaluation strategies. This data-driven approach complements traditional scouting methods and empowers decision-makers with a more comprehensive understanding of the complex factors that contribute to success at the wide receiver position.
3. Skill Diversity
Skill diversity is intrinsically linked to the concept of random NFL wide receiver selection. A random selection process, by its very nature, captures the wide range of skill sets present within the league’s receiving corps. This diversity provides a rich dataset for analysis and allows for a more comprehensive understanding of how different skills contribute to overall receiver performance. Examining this diversity offers valuable insights into player evaluation, team building, and the evolving dynamics of the game.
-
Route Running Prowess
Route running is a fundamental skill for receivers, and a random selection will likely include players with varying levels of proficiency. Some receivers might excel at short, precise routes, while others may be more adept at deep routes requiring speed and precise timing. Analyzing this diversity allows for comparisons between route running styles and their effectiveness in different game situations. For example, a random sample might reveal that receivers with exceptional route running skills outperform those with superior athleticism but less refined technique.
-
Contested Catch Ability
The ability to secure catches in contested situations is a crucial skill for receivers. A random selection process will inevitably include receivers with varying levels of success in contested catch scenarios. Some may excel at high-pointing the ball, while others may possess superior strength and body control to box out defenders. Analyzing catch rates in contested situations within a random sample provides insights into the physical attributes and techniques that contribute to success in these critical moments.
-
Yards After Catch (YAC) Proficiency
Yards after catch represent a receiver’s ability to generate additional yardage after securing the ball. A random sample will include players with diverse YAC abilities, some excelling at elusiveness and agility, others relying on speed and power. Analyzing YAC production within a random sample allows for comparisons between different playing styles and their impact on offensive efficiency. This data can be used to evaluate the effectiveness of different coaching strategies and player development programs focused on maximizing YAC potential.
-
Blocking Capabilities
While often overlooked, blocking is an important skill for receivers, contributing to the success of running plays and screen passes. A random selection will include receivers with varying blocking abilities, some demonstrating superior technique and physicality in engaging defenders, others displaying less proficiency in this area. Analyzing the impact of receiver blocking within a random sample can reveal its contribution to overall offensive success. This data can be particularly valuable for teams that emphasize a balanced offensive attack.
By examining skill diversity within a randomly selected group of NFL wide receivers, analysts gain a more holistic understanding of receiver performance. This approach moves beyond singular metrics like total yards or touchdowns, providing a richer perspective on the diverse skill sets that contribute to success at the position. Furthermore, this analysis offers valuable insights into the evolution of the game, as the relative importance of different skills may shift over time due to rule changes, offensive scheme innovations, and the overall competitive landscape of the league. Understanding these shifts in skill valuation is crucial for effective player evaluation, draft strategy, and player development within the NFL.
4. Talent Evaluation
Talent evaluation in the NFL often relies on a combination of subjective scouting reports, objective performance metrics, and combine results. Introducing the element of randomness challenges these traditional methods by providing a broader, less biased perspective. The cause-and-effect relationship is clear: randomly selecting receivers forces evaluators to consider players they might otherwise overlook, thereby potentially uncovering hidden talent or exposing limitations in traditional scouting methods. For instance, a randomly selected group might include a late-round draft pick with exceptional contested catch statistics, prompting further investigation into why this player was initially undervalued. This process can expose biases in scouting reports that overemphasize physical attributes or pedigree over demonstrable on-field performance.
The importance of talent evaluation as a component of analyzing randomly selected receivers lies in its ability to refine and improve existing evaluation processes. By comparing the performance of randomly selected groups against pre-draft projections, teams can identify strengths and weaknesses in their scouting methodologies. For example, if a team consistently undervalues receivers who excel in yards after catch, this insight can inform future draft strategies and player development programs. Furthermore, analyzing randomly selected groups can reveal the predictive value of different metrics. Perhaps a specific combine drill correlates strongly with on-field success for a randomly selected group, suggesting its potential value as a talent evaluation tool.
In conclusion, incorporating randomness into talent evaluation challenges conventional wisdom and encourages a more data-driven approach. While traditional methods remain valuable, embracing randomness provides a crucial counterpoint, promoting objectivity and uncovering hidden insights. This approach requires a willingness to question established practices and embrace the potential for unexpected discoveries. The challenges lie in integrating this approach into existing scouting workflows and interpreting the results within the complex dynamics of the NFL. However, the potential benefits, including improved draft strategies, more effective player development, and a deeper understanding of receiver performance, make the pursuit of randomness a valuable endeavor for any team seeking a competitive edge.
5. Objective Comparison
Objective comparison forms a cornerstone of analysis involving randomly selected NFL wide receivers. Random selection, by its very nature, minimizes bias and allows for comparisons based on performance data rather than preconceived notions about player reputation or team affiliation. The cause-and-effect relationship is clear: random selection creates a diverse and unbiased sample, enabling objective comparisons that can challenge conventional wisdom and reveal hidden trends. For instance, comparing the catch rates of a randomly selected group of receivers against league averages might reveal an undrafted free agent performing at a level comparable to a high-profile first-round pick. This objective comparison, facilitated by random selection, provides a data-driven perspective that transcends subjective evaluations based on draft position or perceived potential.
The importance of objective comparison as a component of analyzing randomly selected receivers lies in its ability to expose flaws in traditional evaluation methods and uncover undervalued talent. By comparing performance metrics across a diverse, randomly selected group, analysts can identify outliers and gain a deeper understanding of the factors that contribute to success at the receiver position. For example, analyzing the yards-after-catch performance of a random group might reveal that receivers from smaller colleges are just as effective in generating yards after the catch as those from major football programs. This objective comparison challenges the traditional emphasis on pedigree in player evaluation and opens up new avenues for talent identification. Furthermore, objective comparison facilitates a more nuanced understanding of player performance by considering the context of their situation. Comparing a receiver’s performance relative to their team’s overall offensive output provides a more accurate assessment of their individual contribution than simply looking at raw statistics.
In conclusion, objective comparison serves as a crucial tool for analyzing randomly selected NFL wide receivers. It fosters data-driven insights, challenges biases in traditional evaluation methods, and promotes a deeper understanding of receiver performance. The challenges lie in selecting appropriate metrics for comparison and interpreting the results within the complex dynamics of the NFL. However, the potential benefits, including improved talent identification, more effective player development strategies, and a more nuanced understanding of the factors that contribute to success at the wide receiver position, make objective comparison an essential component of any serious analysis involving randomly selected NFL players. This approach ultimately contributes to a more comprehensive and data-driven understanding of the game itself.
Frequently Asked Questions
This section addresses common inquiries regarding the analysis of randomly selected NFL wide receivers. Clarifying these points provides a stronger foundation for understanding the value and implications of this analytical approach.
Question 1: Why analyze randomly selected receivers rather than focusing on top performers?
Analyzing top performers provides insights into peak performance, but studying a random group offers a broader understanding of the league’s receiver talent pool. This approach can reveal hidden trends and challenge conventional wisdom regarding player evaluation.
Question 2: How does randomness ensure objectivity in player evaluation?
Randomness eliminates biases related to reputation, team affiliation, or draft position. It forces consideration of players who might otherwise be overlooked, allowing for a more objective assessment of talent.
Question 3: What statistical methods are most effective for analyzing randomly selected receivers?
Various statistical methods are applicable, including distribution analysis, correlation analysis, regression modeling, and hypothesis testing. The most effective method depends on the specific research question being addressed.
Question 4: Can analyzing randomly selected players truly improve talent evaluation in the NFL?
By challenging traditional scouting methods and revealing hidden trends, this approach can refine evaluation processes. It allows for data-driven insights that complement subjective assessments.
Question 5: How does the concept of skill diversity relate to randomly selected receivers?
Random selection captures the diverse range of skills present within the league. Analyzing this diversity provides insights into the relative importance of different skills and their contribution to overall performance.
Question 6: What are the limitations of analyzing randomly selected receivers?
While valuable, this approach should not replace traditional scouting entirely. Contextual factors, such as team schemes and opponent strength, must also be considered for a complete player evaluation.
Understanding these key questions allows for a more informed interpretation of analytical findings related to randomly selected NFL wide receivers. The insights gained from this approach can significantly contribute to a deeper understanding of player performance and league trends.
The next section will explore specific case studies demonstrating the practical application of analyzing randomly selected NFL wide receivers.
Tips for Analyzing Randomly Selected NFL Wide Receivers
This section provides practical guidance for effectively analyzing data from randomly selected NFL wide receivers. These tips emphasize objective assessment and data-driven insights.
Tip 1: Define Clear Objectives.
Clearly defined research questions are essential. Whether examining the impact of draft position on performance or exploring the correlation between height and contested catch rate, specific objectives guide the analysis and ensure relevant data collection.
Tip 2: Utilize Representative Sample Sizes.
Sample size significantly impacts the reliability of findings. Larger samples generally provide more robust and generalizable results, mitigating the influence of outliers.
Tip 3: Employ Appropriate Statistical Methods.
Different research questions require different statistical tools. Correlation analysis examines relationships between variables, while regression modeling predicts future performance based on various factors. Selecting the right tool is crucial for accurate interpretation.
Tip 4: Consider Contextual Factors.
While random selection promotes objectivity, contextual factors remain important. A receiver’s performance can be influenced by team scheme, opponent strength, and injuries. Integrating these factors into the analysis provides a more complete picture.
Tip 5: Validate Findings with Multiple Data Sources.
Relying on a single data source can introduce bias. Cross-referencing findings with multiple reputable sources strengthens the validity of the analysis and minimizes potential errors.
Tip 6: Document the Methodology Thoroughly.
Transparency is crucial for replicating and validating research. Detailed documentation of the selection process, data sources, and statistical methods enables others to scrutinize and build upon the findings.
Tip 7: Interpret Results Cautiously.
Correlation does not equal causation. While statistical analysis reveals relationships between variables, careful interpretation is necessary to avoid drawing erroneous conclusions. Consider potential confounding factors and alternative explanations.
Tip 8: Iterate and Refine the Process.
Data analysis is an iterative process. Regularly reviewing and refining the methodology, incorporating new data, and revisiting research questions enhances the accuracy and value of the analysis over time.
By following these tips, analysts can derive meaningful insights from data on randomly selected NFL wide receivers. These data-driven insights can inform talent evaluation, player development, and strategic decision-making within the league.
The following conclusion synthesizes the key takeaways from this exploration of analyzing randomly selected NFL wide receivers.
Conclusion
Analysis of randomly selected NFL wide receivers offers valuable insights into player performance, talent evaluation, and league trends. This approach, emphasizing data-driven objectivity, challenges traditional scouting methods and encourages a more nuanced understanding of the wide receiver position. Key takeaways include the importance of unpredictability in sample selection, the power of statistical analysis in revealing hidden patterns, and the significance of skill diversity in evaluating player contributions. Objective comparison, facilitated by random selection, enables unbiased assessment of talent and exposes potential flaws in conventional evaluation processes. Furthermore, understanding the relationship between random player selection and talent evaluation offers opportunities to refine scouting strategies and improve player development programs.
The exploration of randomly selected NFL wide receivers presents a pathway toward a more data-driven and objective understanding of the game. Further research and refinement of analytical techniques promise to unlock even deeper insights, ultimately shaping the future of player evaluation, team building, and the evolution of professional football. The challenge lies not in embracing the randomness itself, but in effectively interpreting its results within the complex and ever-changing landscape of the NFL. This pursuit of objective, data-driven analysis holds the potential to revolutionize how talent is identified, evaluated, and developed within the league.
Youtube Video:
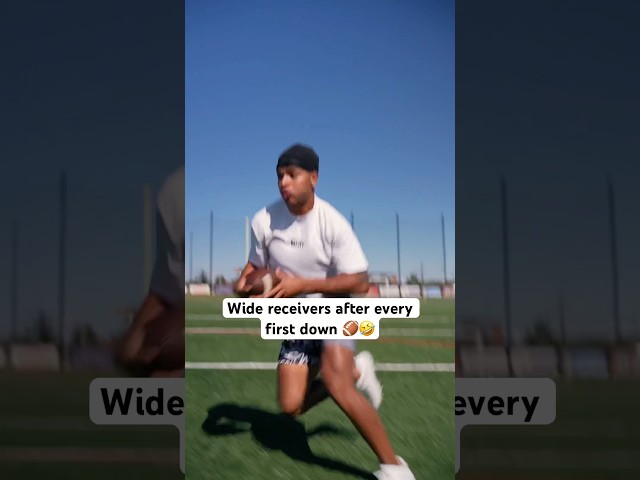